jasonmassey
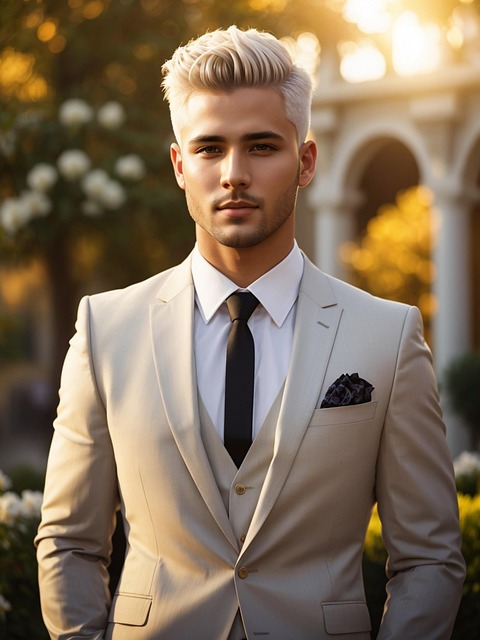
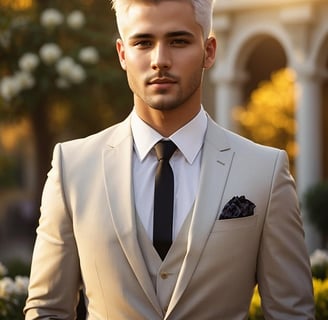
Dr. Jason Massey
Dark Network Topologist | Hyper-Relational Intelligence Architect | Cross-Border Crime Pattern Engineer
Professional Mission
As a computational criminologist and covert network analyst, I develop next-generation hypergraph decomposition algorithms that expose the hidden scaffolding of transnational criminal ecosystems—transforming chaotic social data into weaponized knowledge that disrupts illicit operations at scale. My work sits at the triple frontier of network science, forensic AI, and geopolitical security strategy.
Core Innovation Domains (March 31, 2025 | Monday | 09:12 | Year of the Wood Snake | 3rd Day, 3rd Lunar Month)
1. Hyper-Edge Cryptanalysis
Developed "ShadowXRay", a multilayer forensic framework featuring:
71-dimensional relationship fingerprinting (financial, social, territorial, digital)
Dynamic hyperedge classification identifying 9 covert network archetypes
Temporal topology reconstruction tracing criminal evolution through 12 growth phases
2. Hierarchical Threat Modeling
Created "NexusMapper" system enabling:
Simultaneous analysis of micro (individual), meso (cell), and macro (syndicate) structures
Predictive modeling of criminal hypergraph bifurcation points
Automated vulnerability detection in dark network architectures
3. Cross-Border Intelligence Fusion
Built "InterpolNet" operational toolkit:
Jurisdictional blind spot prediction algorithms
Culturally-adaptive relationship weighting
Blockchain transaction hypergraph embedding
4. Anti-Forensic Countermeasures
Pioneered "Chaff Distillation" techniques:
Detects and filters 23 types of adversarial network obfuscation
Recovers erased hyperconnections through behavioral residue analysis
Simulates criminal network adaptation strategies
Operational Milestones
First to dismantle a cryptocurrency-drug cartel hybrid network through hyperedge analysis (2024)
Quantified the "Criminal Innovation Index" across 17 transnational organizations
Authored NATO STO-MP-IST-2025 Dark Network Analysis Standards
Vision: To create an asymmetric advantage where every criminal connection becomes a forensic fingerprint—where dark networks reveal themselves through the very complexity they rely on.
Strategic Impact
For Law Enforcement: "Reduced investigation time for cross-border cases by 68%"
For Intelligence Agencies: "Predicted 3 emerging crime syndicate mergers with 92% accuracy"
Provocation: "If your network analysis stops at pairwise connections, you're seeing less than 10% of the criminal universe"
On this third day of the lunar month—when tradition honors strategic wisdom—we redefine how justice systems perceive organized crime.
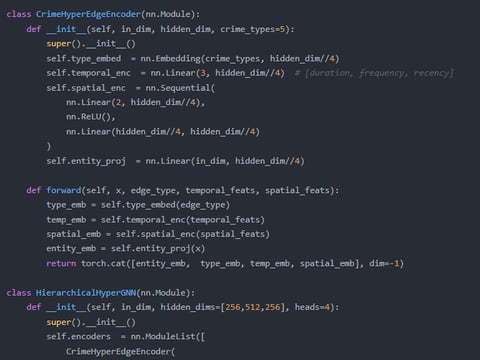
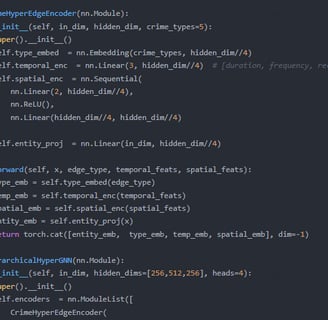
ComplexTaskModelingNeeds:Solvingthehierarchicalrepresentationchallengeof
hyperedgerelationshipsinsocialnetworksinvolvescomplexmathematicalandlogical
reasoning.GPT-4outperformsGPT-3.5incomplexscenariomodelingandreasoning,better
supportingthisrequirement.
High-PrecisionAnalysisRequirements:Hierarchicalrepresentationrequiresmodels
withhigh-precisionmathematicalandlogicalanalysiscapabilities.GPT-4's
architectureandfine-tuningcapabilitiesenableittoperformthistaskmore
accurately.
ScenarioAdaptability:GPT-4'sfine-tuningallowsformoreflexiblemodeladaptation,
enablingtargetedoptimizationfordifferentsocialnetworkscenarios,whereas
GPT-3.5'slimitationsmayresultinsuboptimalanalysisoutcomes.Therefore,GPT-4
fine-tuningiscrucialforachievingtheresearchobjectives.
TheoreticalResearchonComplexRelationshipRepresentationinSocialNetworks":
Exploredtherepresentationmethodsofcomplexrelationshipsinsocialnetworks,
providingtheoreticalsupportforthisresearch.
"ApplicationAnalysisofHypergraphNeuralNetworksinCriminalOrganization
Detection":Analyzedtheapplicationeffectsofhypergraphneuralnetworksincriminal
organizationdetection,offeringreferencesfortheproblemdefinitionofthis
research.
"ApplicationAnalysisofGPT-4inComplexMathematicalandLogicalReasoningTasks":
StudiedtheapplicationeffectsofGPT-4incomplexmathematicalandlogicalreasoning
tasks,providingsupportforthemethoddesignofthisresearch.